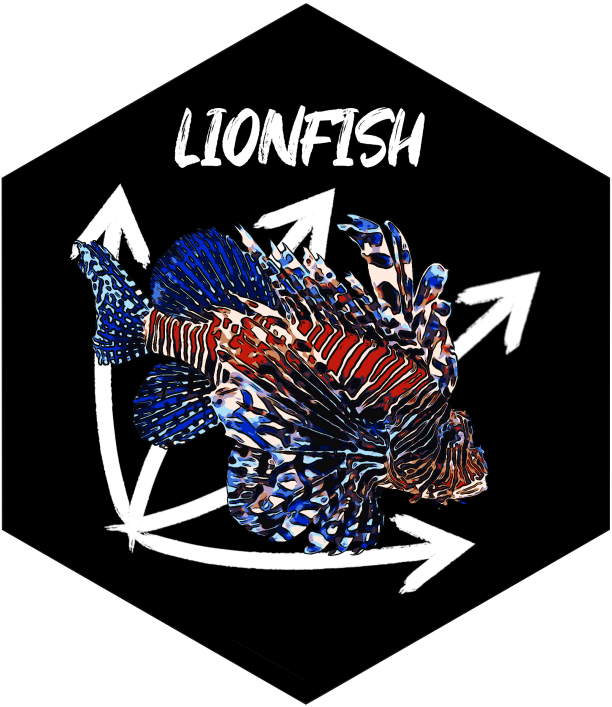
R Wrapper for 'interactive_tour' Function Written in 'python'
interactive_tour.Rd
Launches the lionfish GUI and at minimum requires the data do be loaded and the plot_objects. The other parameters are optional. For technical reasons the parameters half_range, n_plot_cols, n_subsets, color_scale, label_size and display_size cannot be adjusted from within the GUI. The GUI has to be closed and relaunched (possibly with load_interactive_tour) if you want to change them. Please visit https://mmedl94.github.io/lionfish/index.html for a detailed description of the GUI and its features.
Usage
interactive_tour(
data,
plot_objects,
feature_names = NULL,
half_range = NULL,
n_plot_cols = 2,
preselection = FALSE,
preselection_names = FALSE,
n_subsets = 3,
display_size = 5,
hover_cutoff = 10,
label_size = 15,
color_scale = "default",
color_scale_heatmap = "default",
axes_blendout_threshhold = 1
)
Arguments
- data
the dataset you want to investigate
- plot_objects
a named list of objects you want to be displayed. Each entry requires a definition of the type of display and a specification of what should be plotted.
- feature_names
names of the features of the dataset
- half_range
factor that influences the scaling of the displayed tour plots. Small values lead to more spread out datapoints (that might not fit the plotting area), while large values lead to the data being more compact. If not provided a good estimate will be calculated and used.
- n_plot_cols
specifies the number of columns of the grid of the final display.
- preselection
a vector that specifies in which subset each datapoint should be put initially.
- preselection_names
a vector that specifies the names of the preselection subsets
- n_subsets
the total number of available subsets.
- display_size
rough size of each subplot in inches
- hover_cutoff
number of features at which the switch from intransparent to transparent labels that can be hovered over to make them intransparent occurs
- label_size
size of the labels of the feature names of 1d and 2d tours
- color_scale
a viridis/matplotlib colormap to define the color scheme of the subgroups
- color_scale_heatmap
a viridis/matplotlib colormap to define the color scheme of the heatmap
- axes_blendout_threshhold
initial value of the threshold for blending out projection axes with a smaller length
Examples
library(tourr)
data("flea", package = "tourr")
data <- flea[1:6]
clusters <- as.numeric(flea$species)
flea_subspecies <- unique(flea$species)
feature_names <- colnames(data)
guided_tour_history <- tourr::save_history(data,
tour_path = tourr::guided_tour(holes())
)
#> Converting input data to the required matrix format.
#> Target: 1.075, 18.4% better
#> Target: 1.129, 5.0% better
#> Target: 1.159, 2.6% better
#> Target: 1.169, 0.9% better
#> Target: 1.185, 1.4% better
#> Target: 1.193, 0.6% better
#> Target: 1.206, 1.1% better
#> Target: 1.229, 1.9% better
#> Target: 1.233, 0.3% better
#> Target: 1.243, 0.8% better
#> Target: 1.252, 0.7% better
#> Target: 1.257, 0.4% better
#> Target: 1.262, 0.4% better
#> Target: 1.264, 0.2% better
#> Target: 1.269, 0.4% better
#> Target: 1.273, 0.3% better
#> Target: 1.274, 0.1% better
#> Target: 1.276, 0.1% better
#> Target: 1.278, 0.1% better
#> No better bases found after 25 tries. Giving up.
#> Final projection:
#> -0.459 0.478
#> -0.665 -0.152
#> -0.361 -0.263
#> -0.272 -0.540
#> -0.377 0.384
#> -0.044 -0.491
grand_tour_history_1d <- tourr::save_history(data,
tour_path = tourr::grand_tour(d = 1)
)
#> Converting input data to the required matrix format.
half_range <- max(sqrt(rowSums(data^2)))
obj1 <- list(type = "2d_tour", obj = guided_tour_history)
obj2 <- list(type = "1d_tour", obj = grand_tour_history_1d)
obj3 <- list(type = "scatter", obj = c("tars1", "tars2"))
obj4 <- list(type = "hist", obj = "head")
if (check_venv()){
init_env(env_name = "r-lionfish", virtual_env = "virtual_env")
} else if (check_conda_env()){
init_env(env_name = "r-lionfish", virtual_env = "anaconda")
}
if (interactive()){
interactive_tour(
data = data,
plot_objects = list(obj1, obj2, obj3, obj4),
feature_names = feature_names,
half_range = half_range,
n_plot_cols = 2,
preselection = clusters,
preselection_names = flea_subspecies,
n_subsets = 5,
display_size = 5
)
}